Introduction
Prescription medication
overdose is the fastest growing drug-related problem in the USA. The growing
nature of this problem necessitates the implementation of improved monitoring
strategies for investigating the prevalence and patterns of abuse of specific
medications.
Objectives
Our primary aims were to
assess the possibility of utilizing social media as a resource for automatic
monitoring of prescription medication abuse and to devise an automatic
classification technique that can identify potentially abuse-indicating user
posts.
Methods
We collected Twitter
user posts (tweets) associated with three commonly abused medications (Adderall®,
oxycodone, and quetiapine). We manually annotated 6400 tweets mentioning these
three medications and a control medication (metformin) that is not the subject
of abuse due to its mechanism of action. We performed quantitative and
qualitative analyses of the annotated data to determine whether posts on
Twitter contain signals of prescription medication abuse. Finally, we designed
an automatic supervised classification technique to distinguish posts
containing signals of medication abuse from those that do not and assessed the
utility of Twitter in investigating patterns of abuse over time.
Results
Our analyses show that
clear signals of medication abuse can be drawn from Twitter posts and the
percentage of tweets containing abuse signals are significantly higher for the
three case medications (Adderall®: 23 %, quetiapine:
5.0 %, oxycodone: 12 %) than the proportion for the control
medication (metformin: 0.3 %). Our automatic classification approach
achieves 82 % accuracy overall (medication abuse class recall: 0.51,
precision: 0.41, F measure: 0.46). To illustrate the
utility of automatic classification, we show how the classification data can be
used to analyze abuse patterns over time.
Conclusion
Our study indicates that
social media can be a crucial resource for obtaining abuse-related information
for medications, and that automatic approaches involving supervised
classification and natural language processing hold promises for essential
future monitoring and intervention tasks.
Below: Distributions of abuse/non-abuse tweets for the four drugs. The numbers and percentages of abuse-indicating tweets for each drug are also shown
Below: a Distributions of all collected tweets and automatically detected abuse-indicating tweets for Adderall® and oxycodone and b the proportions of abuse-indicating tweets over the same time periods
Full article at: http://goo.gl/IuWsQn
By: Abeed Sarker,
Karen O’Connor, Rachel Ginn, Matthew Scotch, Karen Smith, Dan Malone, and Graciela Gonzalez
Department of
Biomedical Informatics, Arizona State University, Scottsdale, AZ USA
Center for
Environmental Security, Biodesign Institute, Arizona State University, Tempe,
AZ USA
Rueckert-Hartman
College for Health Professions, Regis University, Denver, CO USA
Department of
Pharmacy Practice and Science, University of Arizona, Tucson, AZ USA
Abeed Sarker, Phone: +1-480-884-0349, Email: ude.usa@rekras.deeba.

More at: https://twitter.com/hiv insight
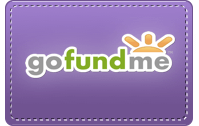
No comments:
Post a Comment