There is significant
heterogeneity within and between populations in their propensity to engage in
conflict. Most research has neglected the role of within-group effects in
social networks in contributing to between-group violence and focused instead
on the precursors and consequences of violence, or on the role of between-group
ties. Here, we explore the role of individual variation and of network
structure within a population in promoting and inhibiting group violence
towards other populations. Motivated by ethnographic observations of collective
behavior in a small-scale society, we describe a model with differentiated
roles for individuals embedded within friendship networks. Using a simple model
based on voting-like dynamics, we explore several strategies for influencing
group-level behavior. When we consider changing population level attitude
changes and introducing control nodes separately, we find that a particularly
effective control strategy relies on exploiting network degree. We also suggest
refinements to our model such as tracking fine-grained information spread
dynamics that can lead to further enrichment in using evolutionary game theory
models for sociological phenomena.
…The process of attitudes spreading across society bears
some resemblance to voter models [26], though the rules
are more complicated. In our multi-scale model, leaders recruit locally,
followed by small friendship-based bursts for collective violence. The overall
social attitude toward participation is shaped by the success or non-success of
these local events. A particularly interesting aspect of the model is that this
recruiting structure appears to be amenable to society-wide interventions that
affect risk-seeking attitudes as well as fine-grained control [27]. While this
framework is motivated by observations of raid recruitment in small-scale
populations [20], it would be
interesting to relax the assumption of local recruitment and consider how
different information flow rules lead to coordination—e.g. when control nodes
(saints/devils) can effectively be “bypassed”. We suggest that this model
framework and the control architectures discussed in this context may be
further investigated in the context of different spreading processes, such as
epidemiological models [28].
Our results may demonstrate the mechanism by which
cultures transition from intergroup violence to peace. Two well-documented
small-scale societies illustrate this process. Until recently, the Enga of New
Guinea and Waorani of Ecuador both had intense warfare. After several decades
of increasingly intense in inter-clan warfare, the Enga have recently
transitioned from a period of chronic warfare to comparatively peaceful
relations due to several factors that parallel our model. First, the death of
many of the most violent individuals known locally as “Rambos” occurred [29] and the population of youth who functioned
as warriors aged out [7]. Second, and more
importantly, communities’ attitudes towards violence have changed towards to
become more pacific, in part because of outside influences and recognition of
the decreasing or marginal benefits from warfare in this case [7]. Among the
Waorani, whose intense cycles of violent revenge threatened the group with
extinction, the process has been similar. Lethal conflicts have been nearly
extinguished through the adoption of cultural values of peace [30] and prominent individuals forgoing
previous norms that would have called for them to engage in violent revenge [31]. This is similar to the processes in our
model where decreasing α (tuning
population-wide attitudes) and having prominent (high-degree) individuals
become “saints” provide a powerful net effect towards the adoption of less
violent action. Our model is illustrative of the dynamics by which these
processes occur, which, at their core, exploit inter-personal influence and
social learning, and applies to decentralized contexts including those of
sectarian, ethnic, or religious conflicts in which there are no state actors
imposing top-down controls. In reality, however, collective non-violence is not
simply the lack of coordinated violence—the path to peace may itself be driven
by its own leaders and follow its own dynamics, which suggests an interesting
extension of adding a competing “pacification process”. And, insofar as the
physical removal (death) of particularly violent individuals (and hence also
their connections) was observed to change the social values towards
non-violence, a further enhancement to the model could include time- or
process-dependent effects such as death. Our framework may also apply to
state-level conflicts that depend on the mobilization of participant support,
and can be integrated into existing frameworks that explore the interaction of
participants and states [32].
Of course, the model is only an approximation to
reality. One aspect that it leaves out is preferential learning, such as one
based more closely on social ties. This may be expected to be particularly
relevant in larger societies where each person only sees a small minority of
all possible people. Such a scenario can give rise to two scales of learning: a
general socially acceptable baseline and much smaller local clusters of more or
less aggressive behavior. Relatedly, this model does not address the social
network evolving in time and can thus be viewed as a relatively short
time-scale model. Expanding the model to more realistically treat large-scale
societies or groups as they evolve over time is a fruitful area of further
research…
Below: Network of connections and designated raid leaders
Full article at: http://goo.gl/sUKIM4
By: Alexander Isakov,#1 Amelia Holcomb,#2 Luke Glowacki,3,4 and Nicholas A. Christakis5,6,7,*
Tobias Preis, Editor
1Department of Physics, Harvard University,
Cambridge, Massachusetts, United States of America
2Department of Mathematics, Yale
University, New Haven, Connecticut, United States of America
3Department of Human Evolutionary Biology,
Harvard University, Cambridge, Massachusetts, United States of America
4Program for Evolutionary Dynamics, Harvard
University, Cambridge, Massachusetts, United States of America
5Department of Sociology, Yale University,
New Haven, Connecticut, United States of America
6Department of Ecology and Evolutionary
Biology, Yale University, New Haven, Connecticut, United States of America
7Yale Institute for Network Science, Yale
University, New Haven, Connecticut, United States of America
University of
Warwick, UNITED KINGDOM
#Contributed equally.
Competing Interests: The authors have declared
that no competing interests exist.
Conceived and designed the experiments: AI AH LG NAC.
Performed the experiments: AH. Analyzed the data: AI AH LG NAC. Wrote the
paper: AI AH LG NAC.
* E-mail: ude.elay@sikatsirhc.salohcin
More at: https://twitter.com/hiv insight
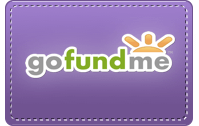
No comments:
Post a Comment